Artificial intelligence offers significant potential for the fintech industry, but success hinges on a strategic approach. The focus should be on maximizing AI’s capabilities while ensuring regulatory compliance, data integrity, and ethical standards. Below, we outline a set of 8 best practices for integrating AI into fintech projects, along with a practical guide to working with Blocshop to bring these strategies to life in your organization.
8 steps to efficient AI implementation in fintech and open banking
1. Identify the right use cases
Effective AI deployment starts with selecting high-impact applications, such as fraud detection, credit risk assessment, and customer personalization. Use cases should provide measurable value, ideally in areas where AI can analyze vast datasets to uncover insights not easily achieved with traditional methods. Focusing on high-value areas ensures resources are used effectively and can produce results that justify further AI investments.
2. Prioritize data governance and quality
AI models thrive on clean, well-organized data. Establishing data governance frameworks is critical, with standards for data integrity, privacy, and quality. This includes defining data sources, managing data access, and ensuring compliance with local and international regulations like GDPR and PSD2. Quality data management improves model accuracy and regulatory adherence.
3. Embed regulatory compliance in AI development
Given the highly regulated nature of fintech, it's important to embed compliance measures throughout AI development. Regulatory checks should be an integral part of each phase, from initial design to deployment. Techniques like model explainability help ensure that AI-driven decisions, especially those affecting credit approval or fraud detection, are transparent and justifiable. This is crucial for meeting regulatory scrutiny.
4. Develop explainability and ethical AI frameworks
Explainable AI (XAI) is essential for building trust, particularly in finance. Models must not only deliver accurate results but also be interpretable, allowing stakeholders to understand how outputs are generated. Ethical frameworks should address algorithmic bias and ensure that AI operates within defined ethical boundaries, especially in sensitive applications like lending or insurance.
5. Start with small-scale, high-impact projects
Begin with pilot projects that are manageable in scope but have the potential for significant business impact. For example, deploying AI in a single department for a specific task, such as automating routine compliance checks, allows organizations to test models under controlled conditions. Early successes can demonstrate value and guide further investment in AI.
6. Establish cross-functional teams for AI projects
Successful AI integration requires collaboration between data scientists, software developers, regulatory experts, and business leaders. Cross-functional teams bring together diverse expertise, ensuring that AI models not only function technically but also align with business objectives and regulatory requirements.
7. Continuous monitoring and model improvement
AI models in fintech are dynamic; they must be continuously monitored for accuracy and adjusted to adapt to new data trends, regulatory changes, or shifts in market behavior. Establish feedback mechanisms to identify when models need retraining and maintain a schedule for periodic updates to keep AI systems robust and relevant.
8. Strengthen cybersecurity protocols
AI adds layers of complexity to existing cybersecurity frameworks. It’s crucial to secure data pipelines, enforce authentication on APIs, and perform regular security audits to address vulnerabilities. A secure AI environment ensures that sensitive data remains protected from emerging threats.
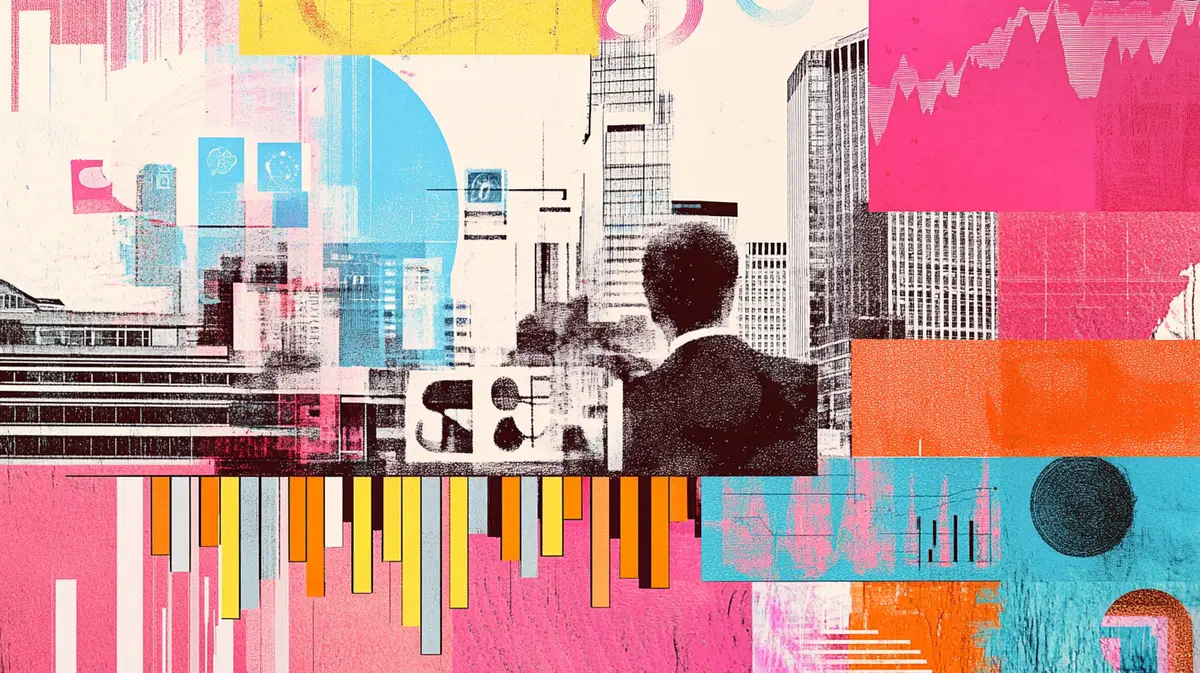
Example: Navigating data fragmentation, compliance, and bias
Implementing AI projects in fintech and open banking comes with a range of challenges, particularly when dealing with complex systems like the UK's pensions dashboard (created to consolidate various pension data sources into a unified view for users, which presents unique data integration challenges for AI). The fragmented nature of pension data—held by different providers, in different formats, and often with varying levels of data quality—makes it difficult to ensure the accuracy and consistency of AI models.
Additionally, complying with evolving regulations, such as GDPR and the Pension Schemes Act, requires careful management of data privacy, access controls, and consent mechanisms. Another challenge is achieving AI explainability in high-stakes areas like credit risk assessments or retirement planning advice, where decisions must be transparent and justifiable to meet regulatory standards. Addressing algorithmic bias is also critical, as financial decisions directly impact users’ lives.
Successfully navigating these issues requires a robust framework for data governance, collaboration across departments, and iterative model development to adapt to new regulations and data sources.
Practical implementation with Blocshop
Blocshop offers the expertise and resources necessary to guide fintech companies through the complexities of AI integration.
Consulting on the use case selection: Blocshop can help organizations identify the most beneficial AI applications by analyzing existing processes and data sources to pinpoint areas with the highest potential for AI-driven improvement.
Data strategy and quality management: With a focus on data governance, Blocshop can develop a framework tailored to an organization's regulatory landscape, enhancing data integrity and ensuring continuous compliance.
AI model development with explainability: Blocshop’s expertise in creating explainable models means clients gain AI solutions that not only perform well but also meet compliance requirements by providing useful results.
Pilot project support: Blocshop facilitates end-to-end pilot project development, helping organizations design, deploy, and evaluate small-scale AI initiatives. Lessons learned can be leveraged to guide broader AI adoption.
Cross-functional team development and training: Blocshop can support fintech companies in setting up collaborative teams with a mix of AI and domain-specific expertise, ensuring sustained project success.
Ongoing monitoring and optimization: Post-deployment, Blocshop can implement monitoring tools and update models to ensure they remain accurate and compliant as conditions evolve.
The combination of these practices provides a solid approach for AI integration in fintech, addressing technical, regulatory, and ethical considerations to ensure sustainable results. At Blocshop, we will help you with audits, analysis, consultations, and practical technical solutions and products that overcome any identified challenges.